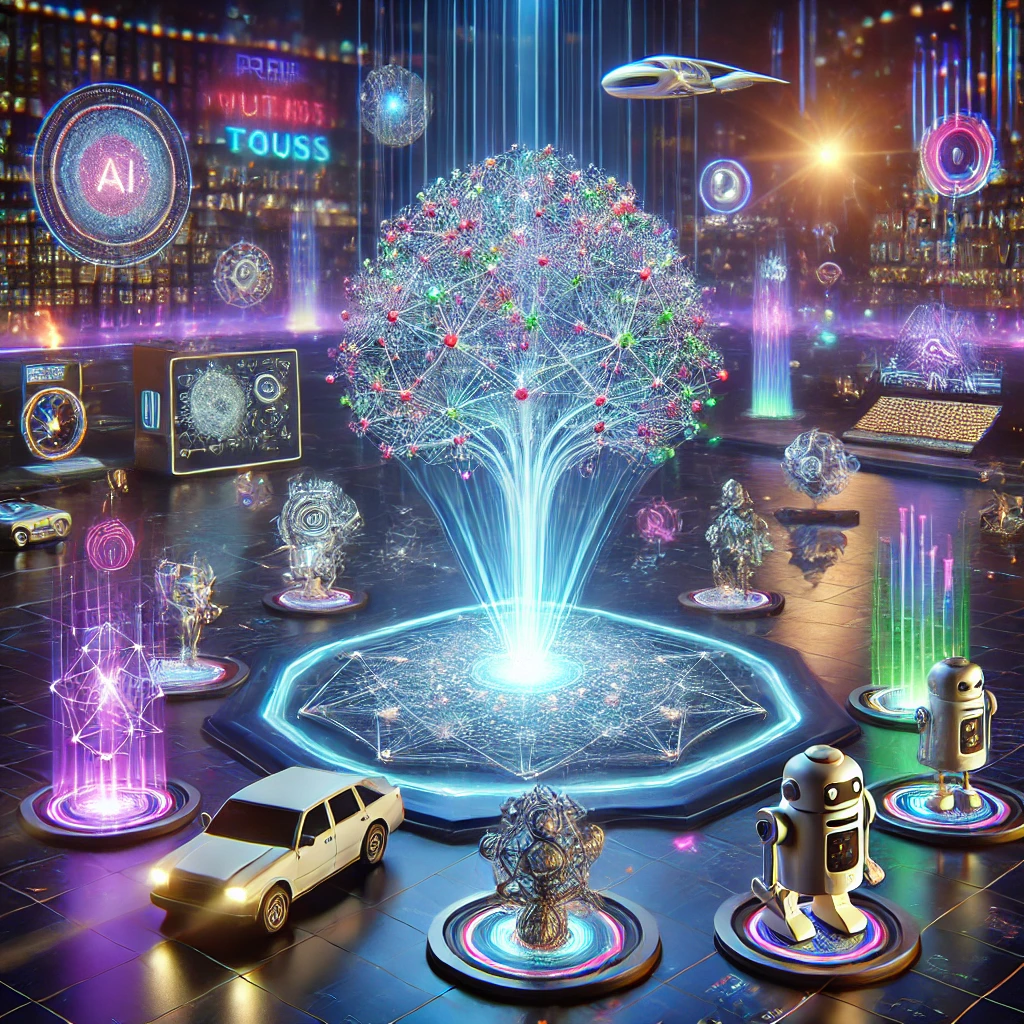
Introduction
Artificial Intelligence (AI) is at the forefront of technological innovation, transforming how we work, communicate, and create. Among the most impressive advancements are pre-trained multi-task generative AI models. These AI models are not just single-purpose tools; they are versatile, robust systems trained to perform multiple tasks without the need for extensive task-specific training. From generating text and images to coding and translating languages, these models are reshaping industries by offering scalable, efficient solutions. This article explores the workings, applications, benefits, challenges, and future potential of pre-trained multi-task generative AI models.
What Are Pre-Trained Multi-Task Generative AI Models?
Pre-trained multi-task generative AI models are sophisticated AI systems designed to handle multiple tasks simultaneously. These models, such as OpenAI’s GPT (Generative Pre-trained Transformer) series, Google’s BERT (Bidirectional Encoder Representations from Transformers), and DALL-E, are trained on vast datasets and fine-tuned to perform various tasks, including text generation, image creation, translation, and more.
Key Features of Pre-Trained Multi-Task Generative AI Models:
- Versatility: These models are capable of performing a wide range of tasks, from content creation to software development, with minimal additional training.
- Generative Capabilities: They can produce new data, such as generating human-like text or creating images from textual descriptions.
- Multi-Task Learning: Unlike traditional models that focus on a single task, these generative AI models can handle multiple functions, making them ideal for various industries.
How Do These Models Work?
- Pre-Training Phase
- During the pre-training phase, the model is exposed to massive amounts of data from sources like books, articles, websites, and code repositories. This process helps the model learn the structure, patterns, and nuances of language and data. For example, GPT-4 by OpenAI is trained on billions of words, enabling it to understand the context and generate coherent, human-like responses.
- Multi-Task Learning
- Multi-task generative AI models are designed to perform various tasks using a single architecture. This capability allows them to write, translate, summarize, code, and even generate images—all from the same core model. This flexibility is what sets them apart from traditional single-task models.
- Fine-Tuning
- After the initial pre-training, these models undergo fine-tuning to enhance their performance on specific tasks. Fine-tuning involves additional training on targeted datasets relevant to the application, such as customer service dialogues, legal documents, or specific coding languages, making the model more accurate and effective.
- Generative Capabilities
- The term “generative” refers to the model’s ability to create new data based on the patterns it has learned during training. For instance, DALL-E can generate images from textual descriptions, enabling creative professionals to bring visual concepts to life without the need for traditional design tools.
Critical Applications of Pre-Trained Multi-Task Generative AI Models
The versatility of these models allows them to be applied across numerous fields, revolutionizing how tasks are approached and executed.
- Content Creation and Writing Assistance
Generative AI models have become essential tools for writers, journalists, and marketers. They assist in drafting articles, generating ideas, creating social media content, and even composing emails. Models like GPT-4 can produce coherent, contextually relevant text, saving time and enhancing creativity.
- Example: Businesses use tools like Jasper AI to generate blog posts, product descriptions, and marketing copy, significantly reducing the workload on human writers.
- Coding and Software Development
AI models like GitHub Copilot, built on GPT, assist developers by suggesting code completions, debugging, and even writing code snippets. This capability accelerates the development process and reduces errors.
- Example: GitHub Copilot provides real-time coding suggestions directly in integrated development environments (IDEs), enhancing productivity for software engineers.
- Language Translation and Summarization
Multi-task generative AI models are widely used in language translation services, making it easier for businesses to operate globally. They also help summarize lengthy documents, allowing professionals to quickly grasp the main points without reading the entire text.
- Example: Tools like DeepL use advanced AI to provide accurate translations and are increasingly being integrated into global business communications.
- Customer Service and Virtual Assistants
AI-powered virtual assistants, such as chatbots and automated customer service agents, use generative models to interact with customers, answer queries, and provide support. This enhances the efficiency of customer service departments and improves user experience.
- Example: Companies use AI chatbots on websites to provide 24/7 support, handling common questions and reducing the need for human intervention. For instance, Zendesk leverages AI to automate ticket responses, streamlining customer interactions.
- Creative Arts and Design
Generative models like DALL-E allow users to create images, illustrations, and designs based on text prompts. This capability is handy for artists, designers, and marketers who need quick visuals tailored to specific needs.
- Example: DALL-E can generate marketing visuals or conceptual art based on simple descriptions, saving time and expanding creative possibilities for professionals.
- Data Analysis and Predictive Modeling
AI models assist in analyzing large datasets, identifying trends, and making predictions. These capabilities are crucial in finance, healthcare, and logistics, where data-driven decisions are essential.
- Example: In finance, generative models help forecast market trends, optimize trading strategies, and analyze risk factors, enhancing decision-making processes.
Benefits of Using Pre-Trained Multi-Task Generative AI Models
- Versatility
- One of the most significant advantages of these models is their ability to perform multiple tasks. Whether writing, coding, translating, or creating visuals, a single model can handle everything, making it highly adaptable.
- Efficiency and Speed
- Pre-trained generative models significantly reduce the time required to complete tasks. Automating repetitive or complex tasks allows professionals to focus on higher-level work that requires human judgment.
- Cost-Effectiveness
- Implementing these models can lead to significant cost savings, especially for businesses. Automating tasks that would otherwise require manual labor reduces operational costs and improves overall productivity.
- Scalability
- These models can quickly scale to meet growing demands. Whether used in customer service or content creation, generative models can handle increasing volumes without additional resources.
- Improved Accuracy and Quality
- Fine-tuning allows these models to provide highly accurate outputs tailored to specific tasks, reducing errors and enhancing the quality of the results.
Challenges and Considerations
Despite their numerous benefits, pre-trained multi-task generative AI models come with challenges that must be addressed.
- Bias and Fairness
- AI models can inherit biases from their training data, leading to biased outputs. It is crucial for developers to continuously monitor and refine these models to ensure fairness and avoid perpetuating harmful stereotypes.
- Data Privacy and Security
- Handling sensitive data with AI models raises concerns about privacy and security. Ensuring compliance with data protection regulations is essential, mainly when used in sectors like healthcare and finance.
- Dependence on Quality Training Data
- The performance of generative models heavily depends on the quality of the data used during training. Poor-quality data can result in accurate and relevant outputs, affecting the model’s reliability.
- Complexity of Integration
- Integrating these advanced models into existing workflows requires significant technical expertise. Businesses may need to invest in training and infrastructure to leverage these AI capabilities fully.
Future of Pre-Trained Multi-Task Generative AI Models
The future of generative AI models is bright, with ongoing research and development aimed at enhancing their capabilities. Upcoming improvements may include:
- Better contextual understanding.
- Emotional intelligence in virtual assistants.
- More refined content generation techniques.
- Better Contextual Understanding: Future AI models will likely understand user context more deeply, leading to more accurate and relevant outputs.
- Emotional Intelligence in Virtual Assistants: As AI evolves, virtual assistants may develop the ability to detect and respond to human emotions, making interactions more natural and empathetic.
- Integration with Emerging Technologies: Expect greater integration of generative AI models with augmented reality (AR) and virtual reality (VR), creating immersive experiences and new possibilities in interactive content creation.
- Ethical and Transparent AI: Efforts to ensure ethical AI practices will drive the evolution of these models, emphasizing transparency, fairness, and responsible use.
Conclusion
Pre-trained multi-task generative AI models are redefining how tasks are performed across various industries. Their versatility, efficiency, and ability to generate high-quality outputs make them invaluable tools in today’s digital world. As these models continue to improve, their impact will only grow, pushing the boundaries of what is possible with AI. Businesses, professionals, and creatives can leverage these powerful models to enhance productivity, innovate processes, and achieve new levels of success.
External Resources and Tools
DALL-E 2: Discover how this AI tool can create unique images from simple text prompts
OpenAI’s GPT: Explore how GPT models are used for text generation, coding, and more.
Can you be more specific about the content of your article? After reading it, I still have some doubts. Hope you can help me.